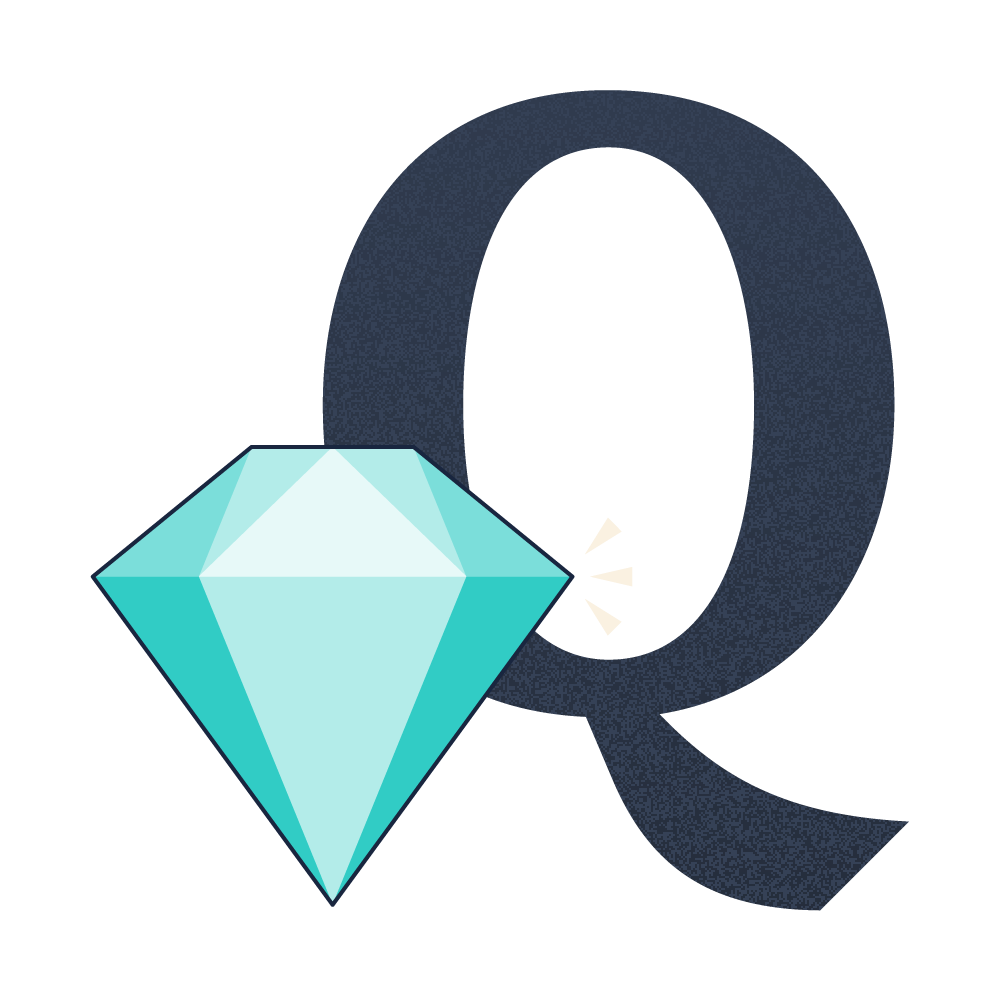
Quality
Data quality is a measure of the condition of data based on factors such as accuracy, completeness, consistency, reliability and whether it's up to date.
Good quality data can drive success in any organization. It’s a valuable asset that allows improved decision-making, leading to improved products, services, customer satisfaction, company reputation, more new customers, and increased profit.
Poor quality data, on the other hand, results in flawed business information, impacting key operational and strategic decisions. Marketing and sales campaigns are less effective and these resources are poorly used. Reports based on the data are inaccurate and unreliable, so creating a single customer view becomes increasingly difficult, processes are fragmented, and money is wasted. This is not a new issue. According to IBM, poor data across businesses and the government costs the US economy $3.1 trillion a year, and Dun & Bradstreet have estimated that the cost of bad data could be as much as 15 to 20% of corporate operating revenues. In addition to the financial wastage, the long-term damage to the brand cannot be underestimated. A more recent study in the UK found over 60% of businesses reporting that their data was out-of-date, incomplete, or missing.
Collecting good quality data is not the end of the story. Data starts to decay from the very moment that it’s collected. Your customers move, change their phone numbers and email addresses; parts and products are given new catalog numbers, stop being manufactured, or are replaced; staff comes and goes. USPS adds or changes over 54,490 addresses daily!
For the best data quality, it's better to collect good data than to correct bad data. Experience suggests that 90% of data quality can be achieved using 10% of the total effort, and this effort is best concentrated at the data entry stage. In fact, often errors created at the data entry or user-interface stage cannot be corrected at all, or only at a significant cost.